BCIN Research Leaders and Research Areas
Shu-Ching Chen, Professor, Multimedia and Disaster Information Management
Steve Luis, IT Director/Business Relations, BCIN Project Manager
Overview
The Business Continuity Information Network (BCIN) addresses the nation’s need to enable community-based disaster data management. Its full deployment will link hundreds of thousands of companies, as well as relevant government agencies, e.g. Emergency Operation Centers, together, thus providing them with the means to share critical recovery information, to assess disaster impacts, to communicate and coordinate recovery efforts, and to locate recovery assistance and resources, as well as with intelligent analytical tools to aid business decision making in face of chaos.
The scale and scope of the problem are challenging. Disaster scenarios affecting hundreds of thousands of companies (from small to large businesses) falling under many different government jurisdictions have been seen in the last several years via the strikes of Hurricanes Katrina, Rita, Wilma, and Ike. How does a business or other organization find the data they need to assess their situation? How do they even know what data they should be looking for? Simply sharing information and making it available is not sufficient. Each organization and business has its own unique needs and is part of its own network of jurisdiction, supply chain, and customer base. The information provided to it must be customized in a way that allows effective decisions to be made under the stress following a disaster – the right data must be given to the right people at the right time.
Research is needed to achieve the scale and provide the needed capabilities:
- The capability to collect, integrate, filter and deliver up-to-the-minute information about infrastructure conditions, situation reports, assistance and recovery efforts, both before and after a disaster, e.g. hurricane, impact. The information is structured, customized and packaged to individual company needs based on their geography and business profile.
- Tools for companies to gather, integrate and report disaster damages to and situation assessments about their companies infrastructure and operations, as well as needs for assistance, both within the company’s organization structure and, if needed, to government agencies, e.g. the Emergency Operation Center.
- Tools for disaster recovery managers to communicate and coordinate (events or actions) both within their company’s organization hierarchy and with external partners or government agencies, as well as to quickly disseminate critical recovery information throughout the supply chain and community (e.g. reopening of ATMs in the disaster region).
- A platform for a multi-tier B2B social network, which includes government, NGOs, and private companies, to assist businesses in obtaining disaster recovery resources and assistance, and a marketplace for companies to share, exchange and market disaster recovery goods and services.
- Intelligent analytical and data mining tools that help decision makers to achieve comprehensive disaster recovery situation awareness, risk assessment, and decision making.
- The data collected and the information created will drive further collaborative research in areas such as supply chain logistics and management, disaster recovery, and social networks.
BCIN’s Approach
The information that businesses and organizations need and can share must be integrated in a way that does not require them to change their existing behavior or data management standards and practices. This means that the system needs to be very flexible in how it can ingest data from existing databases and from data sources such as spreadsheets, text documents, GIS information, etc. It must intelligently understand the data and transform them into actionable information. Businesses do not have time to learn a new system, develop new workflows, and invest money in new practices. The technology bar has to be raised to address these issues in order for any community-based disaster data management system to be widely adopted.
Our research involves academic and industry research communities and domain experts ranging from weather modeling to high-performance computing, to develop technologies that support the first-of-its-kind Business Continuity Information Network, which will help companies form a community that allows them to gather, analyze and communicate real-time business intelligence critical to recovery and resiliency.
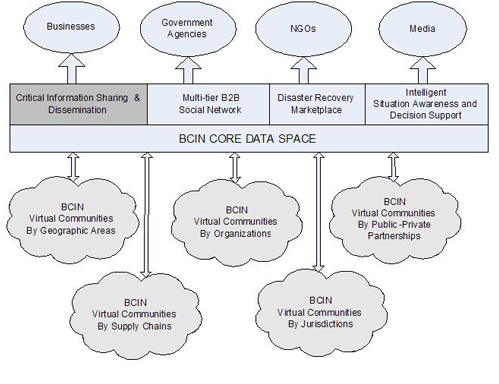
The BCIN project utilizes the latest advances in database integration, data mining, Web, and GIS software technologies to create a user friendly, Internet-based, information-rich service that provides businesses with effective and timely disaster recovery information and that acts as a vital part of a company’s business continuity process, thus potentially reducing closure time. BCIN will allow ad-hoc virtual communities to be built and will provide those communities with tools that: (1) rapidly report, map and share critical up-to-the-minute information about infrastructure conditions and recovery efforts immediately following a storm by working with County Emergency Management Offices and major private infrastructure providers. BCN will localize and tailor relevant information to business managers who will use this information to better assess the hurricane’s impact to their facilities, employees, suppliers and customers; (2) Develop a B2B network, which will include government and NGOs, to assist businesses in creating a disaster recovery marketplace that will allow them to more effectively obtain disaster recovery resources, products, and services while allowing them to collaborate in recovery efforts; (3) Provide a channel for businesses to report their operational status and need of assistance to each other and back to government and NGOs so that these organizations can prioritize their relief efforts and so that decision making can be supported by up-to-the-minute data.
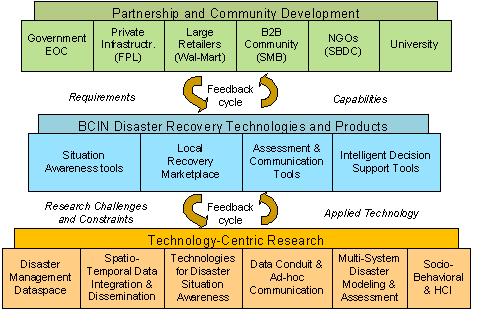
Florida and other hurricane-prone states’ power and communication infrastructure providers have spent hundreds of millions hardening their infrastructure to address the shortfalls exposed by recent storms. A technology solution should expect better power availability and a multitude of communication means in areas that are exposed to hurricane winds, though it may be spotty; even the most devastated areas will be surrounded by communication signals. In addition to always-available satellite-based services, cellular-based data services are inexpensive and widely available through multiple carriers; they provide business owners with an affordable way to keep connected while mobile. Companies like Office Depot encourage their business customers to charge their cell phones and laptops at their stores, and to use their WiFi service for free in the wake of a storm. Hundreds of additional free and commercially-available WiFi communication hotspots are available across regions. This rising availability and resiliency make this the perfect time to leverage information technology to support business resiliency in the face of disasters such as hurricanes.
Disaster Management Dataspace
Faculty collaborators: Drs. Shu-Ching Chen, Evangelos Christidis, and Tao Li (FIU SCIS)
This research component focuses on ways to better organize, ingest, aggregate, and represent streaming and multimedia data relevant to the BCIN ecosystem. This is particularly challenging due to the heterogeneity of the disaster-related data from diverse sources. That is, the disaster-related data can be disaster damage analysis reports and images, open/closure status about roadways/highways/bridges and other infrastructure such as Fuel, Power, Transportation, Emergency Services (Fire Stations, Police Stations etc.), Schools and Hospitals, which may be stored in various formats and can be structured, semi-structured, and unstructured. Providing all the required supporting operations like updates, query, search, and optimization, and accommodating the characteristics like different formats and accessibility through different interfaces, and integration across different administrative and semantic formats, are an existing challenge. All of these call for the needs for creating dynamic virtual communities and for in-depth research on novel transformative methodologies for processing and retrieving information/knowledge from heterogeneous data sources to accommodate the created dynamic virtual communities in a disaster management context.
Toward such needs, in this project, we propose to develop a Disaster Management Dataspace Support Platform (DM-DSSP), with elements suggested by Franklin et al. [FHM05], which improve the DSSP’s general definition to accommodate dynamic virtual communities in a disaster management context. Here, a dynamic virtual community is defined as one of several communities whose members may include one or more companies, government agencies, and other non-governmental organizations which are related by their geographic location, their supply chain dependency, jurisdictional authority, etc. Since a member of each community can participate in multiple communities simultaneously, different relationships can be developed, creating an explosion of interactions which in turn generate a rich set of participant data sets. These communities can be partitioned according to their relative relationships, and thus virtual communities, which are based on their common relational attributes, can be created. Further, new communities appear as a hazard impacts normal operations of a participating member and new relationships are created on-the-fly to respond to recovery needs.
With the increase in popularity of communication via different data types like structured reports, XML documents, unstructured e-mail communications, images, etc., having a common management system for all these data types becomes unavoidable. The concept of dataspace [FHM05] thus became an alternative to traditional Database Management Systems (DBMSs) to handle diverse data types from varied sources. A DSSP can offer a suite of interrelated services on a set of large and loosely coupled data sources, where the data can be under different administrative control and not necessarily under the control of the DSSP, and the relationship between data sources need not be necessarily known. A DSSP provides data integration over all data sources, including those with different levels of complexity and based on different models. It facilitates a uniform interface that examines all available data to provide incremental integration improvement, and searches data sources to help users automatically develop data relationships [FHM05]. It has been shown that a dynamic disaster management system [SLD+08] has the potential of being managed best by a loosely coupled, flexible, dynamic dataspace system as there is a constant influx of information, consisting of structured and unstructured data, of different types and from independent sources, which need to be efficiently stored, indexed, retrieved, and optimized. However, the current DSSP approach requires manually building relationships/partitioning data, which can be referred to as the “man-in-the-loop” problem; this limitation makes it inadequate for our purposes. Therefore, in this research, we exploit the limited scope of the disaster management domain to refine and extend the dataspace approach by developing novel and transformative methodologies to support DM-DSSP so that it can be applied to a disaster scenario, and can provide rapid on-demand data integration within the disaster context tailored for virtual communities (social networking, communities of interest). The success of DM-DSSP will have profound impacts to many research areas (such as data management, data integration, data mining, and information retrieval) and applications (such as digital government, social networks, homeland security, and military).
Our approach distinguishes itself by significantly extending traditional database capabilities and DSSP service guarantees for search, query, and data participant relationship discovery. Our testbed for this effort is a growing network of businesses and county emergency management offices who are sharing disaster management data/information through our existing project the Business Continuity Information Network (BCIN). This first-of-its-kind network is unique in capturing disaster preparation, response and recovery data between communities that must work closely together in order to achieve a speedy recovery of locally impacted economies; this makes BCIN a B2B social network. A large part of disaster preparation, response, and recovery entails government agencies and non-governmental organizations playing specific roles to position and provide resources necessary to restore infrastructure and services needed by the business community and the broader public to maintain operations. Therefore, the dynamic virtual communities are created and depreciated as a normal state for BCIN operations. BCIN further facilitates these processes by providing a unique emergency platform for local businesses to exchange information about available resources, key contacts, threat and recovery situational information, and special needs. Research in social networks such as Betweenness Centrality [Bra01, WB94], Approximation of Between Centrality to reduce computation overhead [GSS08], Visualizing proximity data [DBR+07], Notion of Position in Social Network graphs [BE92], and Regular Equivalence in Social Networks [BE93] are important issues but are not the main focus of this project since this project focuses on leveraging the dynamic virtual communities to create the DM-DSSP to meet the aforementioned goals.
We intend to publish dataspace services to provide researchers in the disaster management community with a valuable research tool. The generalization of our transformative approach can be applied to a wide range of domain areas requiring data exploration tools that take advantage of accumulated knowledge about the user, their relationships, and data they share in various contexts.
Technologies for Disaster Situation Awareness
Faculty Collaborators: Drs. Evangelos Christidis, Tao Li (FIU SCIS)
This research component provides sophisticated disaster situation awareness for the users of BCIN through advanced domain-specific data mining and knowledge discovery technologies. Our context modeling scenario is unique given the richness of input information ranging from data submitted by users of unknown trustworthiness to spatiotemporal association and domain expert wisdom. Querying the Disaster Management Dataspace requires departing from traditional keyword search approaches on static databases and moving to uncertain and multi-modal streaming sources. Combining those sources with the spatio-temporal data sources and mining trends requires novel and domain-specific definitions of item frequencies and patterns. This research is unique, state-of-the-art and transformative to the field. The following outlines the research in this component.
Data Delivery and Semi-automatic Question-Answering on Disaster Dataspace. FIU has been working with IBM TJ Watson [Christidis-et-al-07] on algorithms to generate results in real-time that combine multiple text streams. They have also developed novel collaborative filtering algorithms [Ding-et-al-07] utilizing the intrinsic structures of item similarities. FIU will build a matching mechanism between profiles and data items for a given context, by employing machine learning techniques to measure the semantic distance between business profiles and data items related to disaster management. Historical data will be used as the training set. Traditional machine learning techniques are not adequate since they focus on tabular or plaintext data. In contrast, we need to match the complex business profiles with interaction sequences to streaming structured and unstructured data items. The novel domain-specific machine learning techniques that match profiles to data items will be enhanced by collaborative filtering methods. In addition to the information recommendation mechanism, BCIN will allow stakeholders to submit continuous or ad-hoc information discovery queries on the streaming and stored data. To support ad-hoc or continuous queries of business and government users on the streaming disaster data, effective and efficient information discovery algorithms must be created that take into consideration the context and the stakeholder’s profile. In addition to plain keyword queries, predefined questions (e.g., “is it safe to open my business?”) will be supported, whose answer is computed by appropriately combined rules specified by a domain expert and knowledge learned from analyzing past disaster data (see below). The answer to the queries will be presented using a combination of ranking and navigation techniques, topics on which the PIs have recent SIGMOD publications, exploiting the profile and context information.
Analyze and Learn from the Disaster Management Dataspace. FIU has developed algorithms for discovering pair-wise temporal patterns with predefined time windows [Li&Ma-04]. In [Peng-et-al-07], they developed a novel approach, called event summarization, towards the understanding of the seemingly chaotic temporal data. This component will focus on: (i) Discovering Significant Temporal/Spatial Patterns. In disaster management applications, the patterns they are interested in are location-related business-related temporal/spatial patterns. Correctly understanding and interpreting discovered patterns is also a major challenge. The extracted patterns are generally of large volume and hard to interpret. Building on their existing work, they plan to develop novel techniques for temporal/spatial pattern discovery with semantic awareness. (ii) Identifying Factors Affecting the Recovery. In FIU’s BCIN application, huge numbers of data points will be collected during the recovery process. Some data contain little information related to the recovery, and some are overlapping. It is critical to filter out the less important measures or noises and focus on the small number of measures in a particular context that yield the greatest value. FIU plans to first use unsupervised filter techniques and then explore the correlations among the reduced set of measures by considering the time-shifts. (iii) Analyzing Trend/Phase. It is important to understand the pace/trend of recovery and to divide the recovery process into different stages where each stage has its own characteristics and can adopt different policies and rules. Clustering techniques can be used to automatically divide the recovery data into different clusters that naturally correspond to different phases. One challenge is how to incorporate domain-specific information into the clustering process. For trend analysis, FIU plans to develop semantic-aware clustering techniques for capturing changes and trends. For rare event detection, they will use a local neighborhood which contains both spatial and temporal information to measure the event interestingness and to define semantic deviations.
Localized Modeling and Assessment
Faculty collaborators: S.-Masoud Sadjadi (FIU SCIS), and Patrick Welsh (UNF).
This research component covers hurricane modeling and high-performance computing. Effective disaster management and recovery is a difficult task owing to several complicating factors such as imperfect situation awareness, uncorrelated decision making, and unintended consequences of interactions between complex systems. It is critical for decision makers to understand the inter-correlation of natural, engineering, and social systems.in particular, how these systems respond to disaster events as a whole.in order to develop a comprehensive solution to help the business community to prepare for and rapidly recover from hurricane impacts. This research focuses on multi-disciplinary analysis by coordinating existing complex models and detailed simulations. Specifically, FIU will focus on integrating models for hurricane forecasts for scenario analysis and decision support. FIU.s businesses partners such as Ryder and Greyhound have interest in such tools.
On-demand, multi-scale, and localized hurricane modeling. National Weather Service offices (NWS WFOs) and the National Hurricane Center (NHC) use tools to better understand large-scale weather behavior; however, much of the work to produce neighborhood scale forecasts is done by the NWS meteorologist with larger scale guidance. FIU will provide its NWS partners with higher resolution results so that their National Digital Forecast Database (NDFD) will be able to address issues such as what will happen to my business, my neighborhood, my city, etc., which require high-resolution forecasts. In turn, FIU will ingest the official NDFD forecasts into its business recovery database. Leveraging their existing research in transparent Grid-enablement of scientific applications [Sadjadi-et-al-07], they will develop a distributed software model that can run on computing equipment at multiple sites simultaneously to improve the speed of results, resolution of the numerical model, and at times of hurricane landfall, handle numerous requests by interested parties. This model has the potential to give local WFOs better predictive information for localized areas in Florida.
Other Research Areas
- Security, Privacy, and Trust: In order to make sure the data is secure and also maintain the privacy, research such as role-based access control, privacy-preserving data mining and analysis is needed so that company users are willing to submit and share their information via the BCIN dataspace.
- B2B Social Networks: Research in social networks such as Betweenness Centrality, Approximation of Between Centrality to reduce computation overhead, Visualizing proximity data, Notion of Position in Social Network graphs, and Regular Equivalence in Social Networks are important issues, especially in B2B networks with dynamic virtual communities.
- Mobile Computing and Applications: Most business users would like to use handheld devices to access information in a disaster situation. It is important to study how to make the information available via these devices so that the users can easily find information that is important to their business.
- Web Services and Ontology: By building the BCIN dataspace, BCIN can provide important information and data to its users. Studies are needed on how to provide web services that other applications can leverage to develop other important applications. It is also important to study ontology such as developing a uniform naming catalog for disaster data management, study how to dynamically adapt new categories into this ontology, and investigate how to maintain a consistent naming schema when dealing with communities with conflicting definitions.
- Temporal/Spatial Data Analysis: Disaster information may include temporal and spatial elements. It is very important for the BCIN system to have the capability to process and analyze temporal and spatial data so it can provide the most meaningful information to its users. For the spatial data, BCIN needs to be able to incorporate GIS data so that it has the spatial data management capabilities to collect, process, and provide data/information relevant to a precise location.
- Data Visualization: Research is needed to study what kind of data BCIN should present to its users and how to best present it. Visual information can help the users quickly understand the situation without looking at a large amount of data. Only a limited amount of information can be presented on the small screen of a handheld device, so this is particularly important to mobile users.
- Parallel/Distributed/Cloud Computing: In order to process the large amount of data produced in disaster situations, it is necessary to have enough computing power to meet the load presented by affected users. Studies are needed on how to utilize parallel/distributed/grid/cloud computing to meet the needs of data-intensive computing.
- Multimedia and Multimodal Data Analysis: The disaster data may consist of image, video, and audio such as satellite images and TV News video. We need techniques to process and index these data so that these data can be combined with other data sources to let the users more easily understand their situation.
- Intelligent Information Aging: Studies are needed to study by what factors we age information: Date/time expiration? Based on experience? User-defined aging parameters?
- Human Computer Interaction: Studies are needed to enable users to easily interact with computers and handheld devices so they can easily find the information they need.
- Transportation and Evacuation: Studies are needed on how to evacuate people with cars in light of the limited time frame and existing traffic infrastructure. Research areas such as Intelligent Transportation Systems (ITS) and route guidance are needed.
- User Behavior: Studies of users’ behavior while interacting with the BCIN system are needed. The results of this research will help us to better understand the needs of the users and the strength/weakness of the existing system.
- Social and Economic Impact: Studies of BCIN’s business recovery impact to society and the economy are needed.
- Supply Chain: New methods for managing disaster impacted supply chain response and how to use BCIN as a platform to transmit the real-time status of data whenever available are needed.